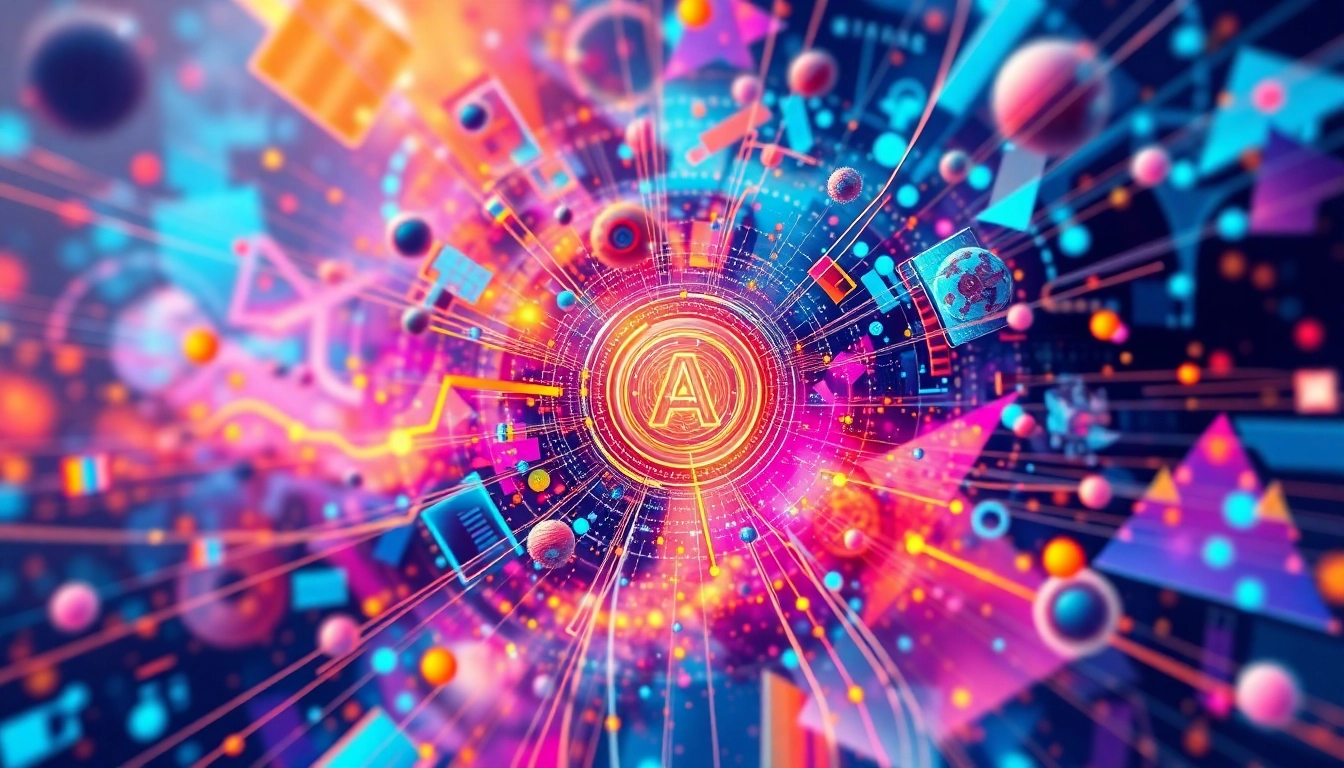
Understanding AI Search Technologies
What is AI Search?
AI search technology represents a sophisticated evolution in the way we retrieve and interact with information online. Unlike traditional search engines that rely primarily on keyword matching and indexing, AI search employs advanced algorithms, including natural language processing (NLP) and machine learning, to provide more accurate, context-aware results. As our reliance on data-driven insights grows, understanding AI search becomes increasingly critical for businesses, researchers, and everyday users alike.
At its core, AI search systems analyze both the intent behind a user’s query and the content of available sources to generate results that are not only relevant but also significantly enriched. This means users are more likely to receive tailored answers that suit their queries, thus streamlining the search process and improving user satisfaction.
How AI Search Differs from Traditional Search Engines
The primary distinction between AI search and conventional search engines lies in the underlying technology and approach to data retrieval. Traditional search engines typically utilize a set of defined algorithms that rank pages based on keyword density, backlinks, and other metrics. In contrast, AI search systems advance beyond simple rankings by leveraging semantic understanding and contextual insights.
For example, AI search engines can understand synonyms, context, and user preferences, allowing them to retrieve and display information more intelligently. This leads to results that are not just based on matching terms but also on comprehending the broader context—essentially anticipating what the user truly seeks. Tools like AI search optimize this process, making information retrieval quicker and more user-centric.
Key Technologies Behind AI Search
AI search relies on several technological advancements to function effectively:
- Natural Language Processing (NLP): This allows machines to understand and respond to human language, interpreting user intent and queries more effectively than traditional keyword-based systems.
- Machine Learning: Algorithms that improve their performance as they process more data, enabling AI search engines to adapt and refine their outputs over time based on user interaction.
- Deep Learning: A subset of machine learning that utilizes neural networks for advanced pattern recognition, critical for tasks like image and voice recognition within search contexts.
- Semantic Search: Understanding the meaning behind queries, ensuring that users receive contextually relevant results rather than just exact keyword matches.
- Data Mining: Extracting patterns from large datasets to allow AI systems to learn from historical behavior and anticipate future queries.
Benefits of Utilizing AI Search
Improved Precision and Relevance
The integration of AI in search processes enhances precision and relevance significantly. Traditional search engines often struggle to understand the nuances of human language, leading to irrelevant results. AI search mitigates this issue by analyzing user queries contextually, delivering results that closely match user intent and requirements. This not only improves satisfaction but also reduces the time spent sifting through irrelevant content.
Enhanced User Experience and Engagement
By delivering highly relevant search outcomes, AI search engines foster a better overall user experience. With fewer irrelevant results to navigate, users can quickly find exactly what they’re looking for. Many AI search platforms also incorporate interactive features such as voice recognition and multi-modal interactions (text, voice, images), further enhancing engagement. A seamless interface helps in retaining users and encourages them to explore more content, ultimately leading to deeper interactions with web properties.
Automation of Information Retrieval
AI search automates the retrieval process, allowing users to obtain answers swiftly without relying on manual research methods. For businesses, this can mean streamlined operations, allowing employees to focus more on value-added tasks rather than spending excessive time on search-related activities. Furthermore, AI search can integrate with various data sources, aggregating information from multiple platforms to present a comprehensive answer to user queries.
Challenges in AI Search Implementation
Data Privacy Concerns
As AI search engines collect and process vast amounts of personal data to deliver customized results, data privacy becomes a paramount concern. Organizations must carefully navigate compliance with regulations such as GDPR, ensuring that user data is handled ethically and transparently. Striking a balance between personalization and privacy will be a critical challenge for businesses leveraging AI search technologies.
Quality of AI-generated Content
AI search engines often produce content based on the patterns and data they have analyzed. While the efficiency of retrieval improves, challenges remain regarding the quality of the output. Users must be wary of misinformation or poorly contextualized information that might be provided. Continuous oversight and quality checks are necessary to maintain the credibility of AI-generated results, as incorrect or biased information can erode trust.
Technical Limitations and Bias
AI systems are not without limitations; they can exhibit biases based on the datasets used to train them. If the training data reflects societal biases, the AI search engine may inadvertently perpetuate these biases in its outputs. Addressing these technical limitations requires ongoing training, updates, and the implementation of fairness metrics in development processes.
Comparison of Leading AI Search Engines
Google Gemini vs. Microsoft Copilot
Two of the most prominent players in the AI search arena are Google Gemini and Microsoft Copilot. Google’s Gemini uses advanced contextual understanding and machine learning capabilities, delivering curated content tailored to user queries. In contrast, Microsoft Copilot integrates deeply with office applications, enhancing productivity by embedding search capabilities directly within workflows.
Both platforms showcase the shift toward integrated, seamless information retrieval experiences, each having its unique strengths. Google’s extensive data and sophisticated algorithms provide a powerful search capability, while Microsoft’s approach focuses on enhancing user productivity in professional environments.
Evaluating User Experiences: Case Studies
To understand the implications of AI search better, it’s essential to evaluate user experiences and case studies across different industries. For instance, a case study of an eCommerce platform utilizing AI search demonstrated a 30% increase in conversion rates due to improved product recommendations that were more aligned with user behavior. This contrasts with traditional search methods, which often yielded a lower relevance of search results.
Furthermore, educational institutions adopting AI search tools report increased engagement among students, who are more likely to explore resources tailored to their interests, thus enhancing learning outcomes. These examples illustrate the significant advantages that AI search can bring across various sectors.
Future Trends in AI Search Technologies
The landscape of AI search is rapidly evolving, with various trends emerging. One significant trend is the shift toward multimodal search capabilities, allowing users to query using text, images, or voice, and receive integrated answers that leverage all available data formats. Companies like Google are investing heavily in this direction, enhancing the capabilities of their search engines to accommodate not just words but the entire spectrum of human expression.
Additionally, the focus on ethical AI will likely bring about new tools and frameworks to ensure that AI search technologies are beneficial to all users. Expect further advancements in explainable AI (XAI) to help users understand how search results were generated, building trust in the process.
Optimizing Strategies for AI Search Integration
Best Practices for Developers and Businesses
Integrating AI search technologies requires a well-thought-out strategy. Key best practices include:
- Understand User Needs: Conduct thorough research to understand what your user base seeks and how they interact with content.
- Invest in Quality Data: Ensure that your training datasets are comprehensive, diverse, and free from biases to construct a powerful AI search engine.
- Iterate and Optimize: Continuously test and refine your search algorithms based on user feedback and performance metrics.
Leveraging AI Search for Competitive Advantage
Businesses can leverage AI search for a significant competitive advantage by focusing on personalization and user engagement. By delivering highly relevant and contextual content, companies not only enhance user satisfaction but also derive actionable insights from user interactions. This cycle of feedback and improvement can propel businesses ahead of competitors who are still reliant on traditional search methods.
Monitoring and Measuring Success in AI Search
Establishing success metrics is crucial in evaluating AI search implementations. Metrics to consider include:
- User Satisfaction: Surveys and feedback loops can measure how users perceive the relevance and accuracy of search results.
- Engagement Metrics: Analyze how users interact with content following a search query, including clicks, time on page, and subsequent actions.
- Conversion Rates: Measure how effectively search queries convert to desired actions, whether that’s a purchase, sign-up, or resource download.
By continually refining search capabilities based on these data points, organizations can ensure their AI search technologies remain effective and user-centric, adjusting to changing trends and expectations in real-time.